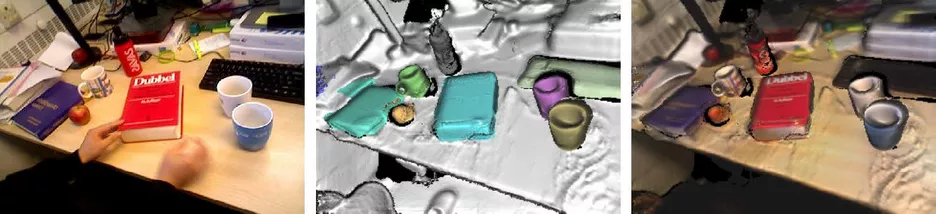
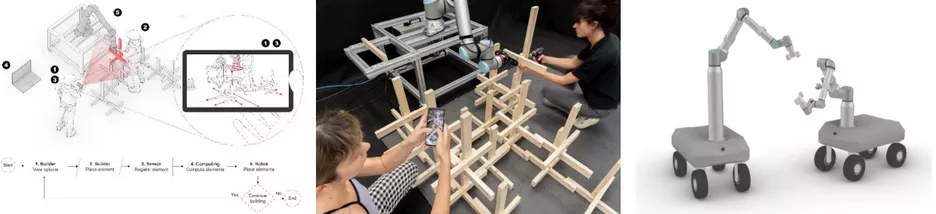
3D Human Motion Capture for Cooperative Construction Robotics
HuMoCap
Abstract
Tracking human motion from a potentially moving camera is crucial for many mobile robotics applications in unstructured environments such as buildings sites that involve deployment amongst humans or even cooperation with humans: robots need to understand human actions, and importantly also acquire the ability to forecast future movements in order to safely avoid collisions and/or carry out tasks collaboratively, possibly in physical interaction. The undertaking is challenging due to (1) ego-motion, (2) the context humans and robots operate in (i.e. 3D and semantic environment as well as tasks), and (3) the lack of readily available, contextualised ground truth data from which to learn data-driven models to allow for more robust tracking and forecasting. This project aims to address all of these by developing an in-the-wild human motion capturing system that leverages visual-inertial Simultaneous Localisation and Mapping (SLAM) for ego-motion estimation as well as dense mapping to provide the geometric and semantic scene context, paired with Deep-Learning-Based human pose estimation and tracking. The data that the system will allow us to collect can later serve as a basis to train advanced motion tracking, forecasting and human-robot collaboration algorithms in the area of cooperative construction robotics.