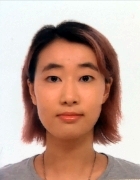

Ayshah Chan
Remote Sensing Technology
Knowledge Distillation from Big Administrative Data (KnowDisBAD)
Data-driven modeling through deep learning techniques has shown tremendous success in various research domains in recent years. However, we require large amounts of high-quality data annotations to train such models. Usually, such data is notoriously scarce and expensive to mine, limiting the translation of machine learning concepts to application domains that seek to benefit from them.
On the other hand, there are huge repositories of information acquired by administrative and governmental bodies to enable public services and processes. Such administrative or government data can be assumed to be acquired according to high-quality standards and in a timely manner. They are usually organized in tabular form, conducive to automated processing. Nevertheless, administrative bodies usually do not follow agreed protocols, making these fragmented data stocks hard to align.
Using the example of farmers’ self-declarations in the context of allocations of agricultural subsidies, the EuroCrops project at the Chair of Remote Sensing Technology seeks to harmonize such data sources and demonstrate its potential. This attempt showed that manual harmonization schemes require significant domain knowledge and many iterations to improve. Such competencies and resources are often unavailable, particularly in a formalized manner. Automated methods for data alignment and harmonization are therefore needed, and recent developments from the machine learning research community, particularly in the field of natural language processing (NLP), can be helpful for this purpose. Recent large language models (LLMs), like the series of Generative Pre-trained Transformer (GPT) models and applications derived from them, such as ChatGPT, successfully extract information from huge and diverse data sources and aggregate it thematically.
Concomitantly, such systems face problems as they risk providing false or misleading information comprehension while appearing overly confident, caused by the uneven and biased distribution of training data and the sheer complexity of the trained models. Therefore, such data aggregation and processing regimes must meet fairness and privacy standards, while explainability and transparency of the trained models need to be ensured.
My project aims to address the above challenges and find answers to the following research questions:
- Can the tedious process of data harmonization be automated, and how does this compare to manually designed harmonizing schemes, e.g., the hierarchical crop and agriculture taxonomy (HCAT) from EuroCrops?
- Can relevant information be identified in harmonized datasets or directly from the raw data sources?
- Can these processes be adapted over time as new data sources are considered?
- How can privacy and fairness concerns be addressed throughout the entire workflow?