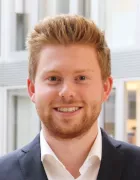

Nils Sturma
Chair of Mathematical Statistics
Identifiability and Inference of Causal Effects in Latent Variable Models
Identifying and quantifying causal relationships from observational data is a central problem in statistics. An intuitive tool for representing causal relationships in a collection of random variables are directed graphs. The nodes correspond to the variables and the directed edges indicate for each variable on which other variables it causally depends. Models corresponding to directed graphs are formalized mathematically by structural causal models which are widely used, for example, in social sciences, economics or genetics.
Often we do not have data for all the variables, and it becomes of interest to determine whether causal effects are uniquely identified on the basis of the observed data only. For example, in the social sciences variables such as "intelligence" or "creativity" are often unobserved (latent) as they cannot be measured directly. Assuming Gaussian noise and linear dependencies between random variables, the linear coefficients represent direct causal effects. Then an effect is identified in a given graph if the corresponding coefficient can be uniquely recovered from the covariance matrix of the associated observable distribution.
In this PhD project we investigate which effects are identifiable in models that explicitly include latent variables. We use algebraic methods such as Gröbner basis computations and aim to develop easily testable graphical conditions for characterizing identifiability. Importantly, we are also interested in the identifiability of causal effects between latent variables, which was not in the focus of related prior research. In a second step we study statistical inference of causal effects by creating estimates based on the observed sample covariance matrix. We make use of the fact that, if identification is possible, causal effects are identified by rational functions in the entries of the covariance matrix of the underlying observable distribution. To facilitate the application of our results, we intend to provide software that decides identifiability in latent variable models and provides consistent estimates for the identifiable causal effects.