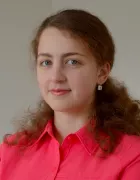

Mariia Gladkova
Computer Vision & Artificial Intelligence
Dynamic Simultaneous Localization and Mapping
In recent years the field of Simultaneous Localization and Mapping (SLAM) has attracted a lot of attention from the research community and companies that are motivated to bring forward the development of autonomous systems such as robots, cars and drones. The core task of SLAM lies in the joint estimation of the robot's location (localization) and construction of a 3D map of the environment it navigates in (mapping). SLAM offers a generalized modular framework that can be adapted to systems with different active and passive sensors, such as cameras, LiDAR, GPS, Radar and others. In particular, cameras are cheap, versatile and lightweight sensors that provide rich and intuitive information in the form of images.
There exist many mature computer vision methods for analyzing visual data and extracting ego-motion of a camera system mounted on a movable platform. Nonetheless, these approaches often rely on the assumption of a static surrounding, therefore they are brittle in the presence of moving objects. To achieve reliable and robust operation in real-world scenarios, it is essential to address this limitation in a systematic manner by integrating object-level information into a SLAM or Visual Odometry (VO) system. To this end, deep learning solutions, which show state-of-the-art performance on various computer vision tasks such as object detection, segmentation and classification, can be leveraged and further adapted to the desired application domain.
As a part of my doctoral studies, I would like to concentrate on combining the detection and tracking of moving objects with classical VO / SLAM methods. The main advantage of such a hybrid system would lie in higher accuracy of camera and objects’ pose estimation along with a consistent reconstruction of a 3D environment. Moreover, in comparison to existing SLAM systems, which treat corresponding dynamic parts of an image as spurious, it is intended to properly model motion of each observed object in the scene and contribute to a richer scene understanding.
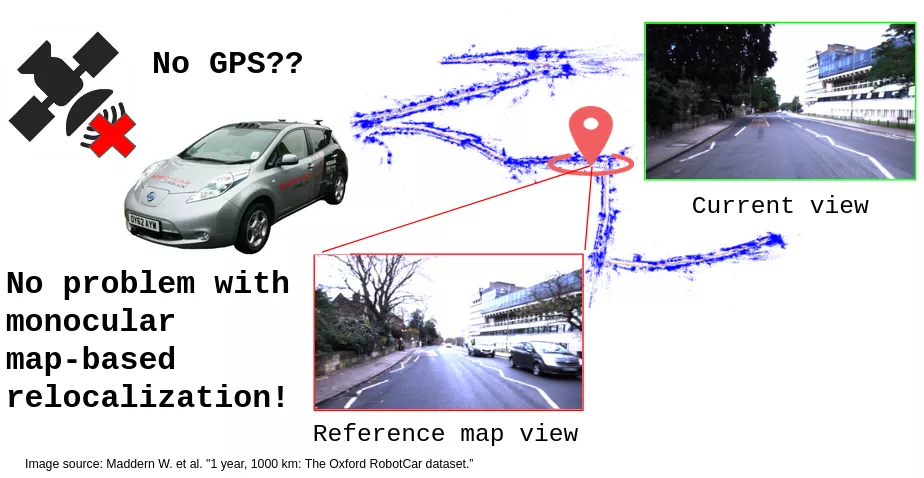
Gladkova M., Wang R., Zeller N., Cremers D. (2021): "Tight Integration of Feature-based Relocalization in Monocular Direct Visual Odometry", Proc. of the IEEE International Conference on Robotics and Automation (ICRA), arXiv:2102.01191