Learning-on-Graphs Conference 2023: Local Meetup
Local Meetup @ Munich
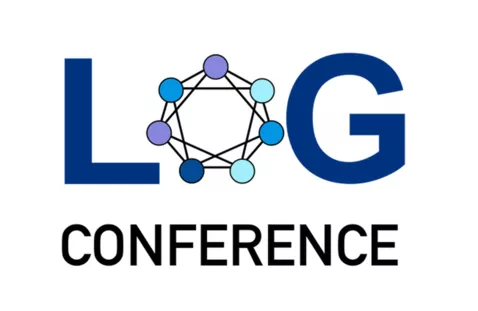
We are happy to have been accepted as a local meetup for the Learning-on-Graphs Conference 2023 and look forward to welcoming you to Munich this autumn! The following information sketches our schedule for a 2-day meetup. At this point, we are at capacity and unfortunately unable to accept further registrations. However, if you would like to join and had your paper accepted at LoG 2023, please reach out to emmelie.korell(at)tum.de.
Dates:
November 30 & December 1, 2023
Schedule:
November 30
Time | Program | Location |
---|---|---|
15:00 | Arrival & Registration | IAS TUM |
16:00 | Orals (Livestream) | IAS TUM |
17:00 | Keynote Prof. Kyle Cranmer (Livestream) | IAS TUM |
18:00 | Tutorials (Livestream) & Networking & Fingerfood | MDSI TUM |
21:00 | Closing Remarks | MDSI TUM |
Dezember 1
Time | Program | Location |
---|---|---|
10:00 | Opening | IAS TUM |
10:15 | Keynote Prof. Christopher Morris Expressivity and Generalization Abilities of Graph Neural Networks | IAS TUM |
11:15 | Talks I | IAS TUM |
12:30 | Lunch Break | MDSI TUM |
13:30 | Panel Discussion | IAS TUM |
14:30 | Talks II | IAS TUM |
15:30 | Poster Session | MDSI TUM |
Attendees are invited to extend their stay for further networking.
Talks
Slot 1: Friday, Dec 1, 11:15-12:30
Name | Talk Title |
---|---|
Alice Moallemy-Oureh | Graph Neural Networks for Attributed Dynamic Graphs in Continuous-Time Representation |
Marco Sälzer | Should We Aim for Sound and Complete Verification for GNN |
Sayed Soroush Haj Zargarbashi | Conformal Prediction for Graph Neural Networks |
Chester Tan | The Map Equation Goes Neural |
Gianluca Galletti | LagrangeBench: A Lagrangian Fluid Mechanics Benchmarking Suite |
Slot 2: Friday, Dec 1, 14:30-15:30
Name | Talk Title |
---|---|
Jan Tönshoff | Where Did the Gap Go? Reassessing LRGB |
Nimrah Mustafa | Are GATs Out of Balance? |
Chirag Varun Shukla | Towards Training GNNs using Explanation Directed Message Passing |
Vincent Grande | Topological Point Cloud Clustering: The Hodge Laplacian and Continuous Representations of Persistent Homology |
Poster
The poster session is scheduled for Friday, Dec 1, 15:30. Posters may be brought, put up & admired already on Thursday afternoon.
Presenters | Poster Title | Authors |
---|---|---|
Jan Schuchardt | (Provable) Adversarial Robustness for Group Equivariant Tasks: Graphs, Point Clouds, Molecules, and More | Jan Schuchardt, Yan Scholten, Stephan Günnemann |
Sohir Maskey | A Fractional Graph Laplacian Approach to Oversmoothing | Sohir Maskey, Raffaele Paolino, Aras Bacho, Gitta Kutyniok |
Artur Toshev | Accelerating Molecular Graph Neural Networks via Knowledge Distillation | Filip Ekström Kelvinius, Dimitar Georgiev, Artur Toshev, Johannes Gasteiger |
Mahalakshmi Sabanayagam | Analysis of Convolutions, Non-linearity and Depth in Graph Neural Networks using Neural Tangent Kernel | Mahalakshmi Sabanayagam, Pascal Esser, Debarghya Ghoshdastidar |
Nimrah Mustafa | Are GATs Out of Balance? | Nimrah Mustafa, Aleksandar Bojchevski, Rebekka Burkholz |
Lorenz Kummer | ATTACKING GRAPH NEURAL NETWORKS WITH BIT FLIPS: WEISFEILER AND LEHMAN GO INDIFFERENT | Lorenz Kummer, Samir Moustafa, Nils Kriege, Wilfried Gansterer |
Vincenzo Perri | Bayesian Detection of Mesoscale Structures in Pathway Data on Graphs | Vincenzo Perri, Luka V. Petrović |
Linlin Jia | Bridging Distinct Spaces in Graph-Based Machine Learning | Linlin Jia, Xiao Ning, Benoit Gaüzère, Paul Honeine, Kaspar Riesen |
Sayed Soroush Haj Zargarbashi | Conformal Prediction for Graph Neural Networks | Soroush H. Zargarbashi, Aleksandar Bojchevski |
Nguyen Khoa Tran | Drug Response Prediction using Multi-Omics Data and Molecular Graphs | Nguyen Khoa Tran, Gunnar W. Klau |
Chendi Qian | Exploring the Power of Graph Neural Networks in Solving Linear Optimization Problems | Chendi Qian, Didier Chételat, Christopher Morris |
Andrei-Dragos Brasoveanu, Pascal Welke | Extending Graph Neural Networks with Global Features | |
Luca Verginer | Forecasting Innovation with GRUs & Patent Embeddings | Luca Verginer |
Patrick Indri | GraphPrivatizer: Improved Structural Differential Privacy for Graph Neural Networks | Rucha Bhalchandra Joshi, Patrick Indri, Subhankar Mishra |
Alice Moallemy-Oureh | Handling of Attribute Dynamics in Graph Learning | Alice Moallemy-Oureh |
Christian Koke | HoloNets: Spectral Convolutions do extend to Directed Graphs | Christian Koke, Daniel Cremers |
Gianluca Galletti | LagrangeBench: A Lagrangian Fluid Mechanics Benchmarking Suite | Artur P. Toshev, Gianluca Galletti, Fabian Fritz, Stefan Adami, Nikolaus A. Adams |
Rebekka Burkholz | Learning on random graphs | Advait Gadhikar, Nimrah Mustafa, Adarsh Jamadandi, Rebekka Burkholz |
Vincent Grande | Non-Isotropic Persistent Homology: Leveraging the Metric Dependency of PH | |
Tamara Drucks | PAN: Expressiveness of GNNs with Paths | Caterina Graziani, Tamara Drucks, Monica Bianchini, Franco Scarselli, Thomas Gärtner |
Andreas Roth | Rank Collapse Causes Over-Smoothing and Over-Correlation in Graph Neural Networks | Andreas Roth, Thomas Liebig |
Jonas Jürß | Recursive Algorithmic Reasoning | Jonas Jürß, Dulhan Jayalath, Petar Velickovic |
Sadegh Akhondzadeh | Rethinking Label Poisoning for GNNs: Pitfalls and Attacks | |
Lukas Gosch | Revisiting Robustness in Graph Machine Learning | Lukas Gosch, Simon Geisler, Daniel Sturm, Stephan Günnemann |
Florian Grötschla | SALSA-CLRS: A Sparse and Scalable Benchmark for Algorithmic Reasoning | Julian Minder, Florian Grötschla, Joel Mathys, Roger Wattenhofer |
Christopher Blöcker | Sampling Networks from Modular Compression of Network Flows | Christopher Blöcker, Jelena Smiljanić, Martin Rosvall, Ingo Scholtes |
Vincent Stimper | SE(3) Equivariant Augmented Coupling Flows | Laurence I Midgley, Vincent Stimper, Javier Antorán, Emile Mathieu, Bernhard Schölkopf, José Miguel Hernández-Lobato |
Marco Sälzer | Sound and Complete Verification of GNN | Marco Sälzer and Martin Lange |
Silvia Beddar-Wiesing | Structural-Dynamic Graph Embedding | Silvia Beddar-Wiesing |
Joël Mathys | SURF: A Generalization Benchmark for GNNs Predicting Fluid Dynamics | Stefan Künzli, Florian Grötschla, Joël Mathys, Roger Wattenhofer |
Chester Tan | The Map Equation Goes Neural | Christopher Blöcker, Chester Tan, Ingo Scholtes |
Moritz Lampert | The Self-loop Paradox: Investigating the Impact of Self-Loops on Graph Neural Networks | Moritz Lampert, Ingo Scholtes |
Leon Klein | Timewarp: Transferable Acceleration of Molecular Dynamics by Learning Time-Coarsened Dynamics | Leon Klein, Andrew Y. K. Foong, Tor Erlend Fjelde, Bruno Mlodozeniec, Marc Brockschmidt, Sebastian Nowozin, Frank Noé, Ryota Tomioka |
Vincent Grande | Topological Point Cloud Clustering: The Hodge Laplacian and Continuous Representations of Persistent Homology | Vincent P. Grande, Michael T. Schaub |
Luis Müller, Frederik Wenkel, Blazej Banaszewski | Towards Foundational Models for Molecular Learning on Large-Scale Multi-Task Datasets | Dominique Beaini, Shenyang Huang, Joao Alex Cunha, Gabriela Moisescu-Pareja, Oleksandr Dymov, Samuel Maddrell-Mander, Callum McLean, Frederik Wenkel, Luis Müller, Jama Hussein Mohamud, Ali Parviz, Michael Craig, Michał Koziarski, Jiarui Lu, Zhaocheng Zhu, Cristian Gabellini, Kerstin Klaser, Josef Dean, Cas Wognum, Maciej Sypetkowski, Guillaume Rabusseau, Reihaneh Rabbany, Jian Tang, Christopher Morris, Mirco Ravanelli, Guy Wolf, Prudencio Tossou, Hadrien Mary, Therence Bois, Andrew Fitzgibbon, Błażej Banaszewski, Chad Martin, Dominic Masters |
Chirag Varun Shukla | Towards Training GNNs using Explanation Directed Message Passing | Valentina Giunchiglia, Chirag Varun Shukla, Guadalupe Gonzalez, Chirag Agarwal |
Tom Wollschläger | Uncertainty Estimation for Molecules | Tom Wollschläger, Nicholas Gao, Betrand Charpentier, Amine Ketata, Stephan Günnemann |
Dominik Fuchsgruber | Uncertainty for Active Learning on Graphs | Dominik Fuchsgruber, Tom Wollschläger, Bertrand Charpentier, Antonio Oroz, Stephan Günnemann |
Franziska Heeg | Using Causality-Aware Graph Neural Networks to Predict Temporal Centralities in Dynamic Graphs | Franziska Heeg, Ingo Scholtes |
Martin Ritzert, Eran Rosenbluth, Jan Tönshoff | Where did the gap go? Reassessing LRGB | Jan Tönshoff, Martin Ritzert, Eran Rosenbluth, and Martin Grohe (both not attending) |
Pascal Welke | Maximally Expressive GNNs for Outerplanar Graphs |
Location: IAS & MDSI

The meetup takes place at TUM Institute for Advanced Studies (IAS) and TUM Munich Data Science Institue (MDSI).
IAS: | MDSI |
---|---|
Lichtenbergstraße 2 a 85748 Garching Germany | Walther-von-Dyck-Str. 10 |
Organizers
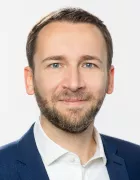
Stephan Günnemann
Technische Universität München
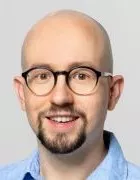
Bastian Rieck
Helmholtz Munich
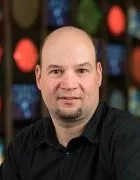
Ingo Scholtes
Julius-Maximilians-Universität Würzburg
Related Research: InterConnect
Graph neural networks and topological data analysis provide strong potential for gaining deeper insights into global patterns in graph-structured data, utilizing a combination of ML, combinatorics, geometry, algebra, and statistics techniques. Studying these networks’ connectivity provides insights into information flow, social dynamics, disease spread, traffic optimization, network resilience, and much more.
Closely related to the concerns of the Learning on Graphs conference, the new MDSI focus topic InterConnect: Interdisciplinary Research on Graphs, Networks, and Connectivity Structures tackles similar topics. If you are interested, please take a look at the focus topic!