The promotion of future data scientists and interdisciplinary experts in a broad spectrum of research areas is deeply rooted in the MDSI's mission. This aims to prepare a new generation of data analysts for the needs of increasingly digitized workflows and value chains beyond academia. With a strong focus on supporting female students, which ties nicely into its efforts to promote diversity in data science, MDSI has established a program for outstanding doctoral researchers in the related science branches.
The MDSI Doctoral Fellowships are an essential component of this program. Their call for applications opens annually and invites doctoral researchers aspiring to start their doctoral project with an MDSI Core Member or a TUM-based ELLIS-Munich member. The successful candidates receive funding for three years, and MDSI also covers a conference trip. Additionally, they profit from the specially tailored MDSI Doctoral Training Program. Furthermore, MDSI strongly supports their collaboration and interaction with fellows and faculty from other research areas and establishes contacts with industry partners.
Until 2025, many doctoral fellowships were financed by our partner Linde. Their funding was aimed in particular at supporting outstanding female doctoral students.
Call for Applications
Please look at the details of the application requirements and the next call for applications.
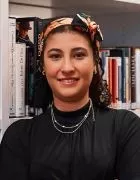
Earth System Modelling
Hybrid Vegetation Modelling
Supervisor: Prof. Dr. Niklas Boers
Supported by: Linde
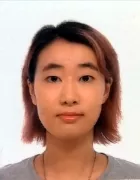
Remote Sensing Technology
Knowledge Distillation from Big Administrative Data (KnowDisBAD)
Supervisor: Prof. Dr. Marco Körner
Supported by: Linde
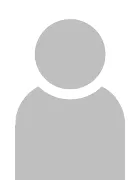
Martin Großhauser
Supervisor: Prof. Dr. Gil Westmeyer
Supported by: Linde
Business Analytics & Intelligent Systems
Scalable Reinforcement Learning for Industrial Applications
Supervisor: Prof. Dr. Maximilian Schiffer
Supported by: MDSI
Artificial Intelligence in Healthcare and Medicine
Supervisor: Prof. Dr. Daniel Rückert
Supported by: Linde
Data Analytics and Machine Learning
Molecular Modelling Based on Neural Networks with Long-Range Methods from Physics, Chemistry, and Materials Science
Supervisor: Prof. Dr. Stephan Günnemann
Supported by: MDSI
Electrochemical Materials Lab
Materials Representation Learning for Synthesis Planning
Supervisor: Prof. Dr. Jennifer Rupp
Supported by: MDSI
Isabel Schorr
Human-Centered Technologies for Learning
Supervisor: Prof. Dr. Enkelejda Kasneci
Supported by: MDSI
Chair of Sustainability Assessment of Food & Agricultural Systems
Integrating Deep Learning and Satellite Imagery for Biodiversity Assessment
Supervisor: Prof. Dr. Livia Cabernard
Supported by: Linde
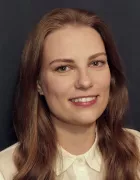
Data Science in Systems Biology
A joint framework integrating microRNA and transcription factor regulation analysis at bulk and single-cell level
Supervisor: Prof. Dr. Markus List
Supported by: Linde
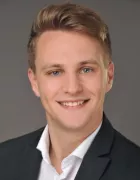
Thermo-Fluid Dynamics
Physics Augmented Machine Learning for Thermoacoustic Modeling
Supervisor: Prof. Wolfgang Polifke, Ph.D.
Supported by: Linde
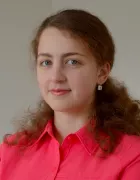
Computer Vision & Artificial Intelligence
Dynamic Simultaneous Localization and Mapping
Supervisor: Prof. Dr. Daniel Cremers
Supported by: Linde
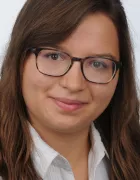
Dynamical Systems
Multiscale Dynamics of Deep Neural Networks
Supervisor: Prof. Christian Kühn, PhD
Supported by: Linde
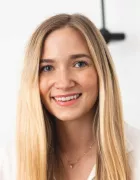
Kristina Mach
Computer Aided Medical Procedures
Computer Vision for Ophthalmologic Surgery
Supervisor: Prof. Dr. Nassir Navab
Supported by: Linde
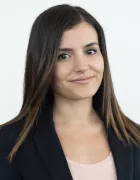
Shiva Madadkhani
Resource Economics
Renewable Energy Economics
Supervisor: Prof. Svetlana Ikonnikova, PhD
Supported by: Linde
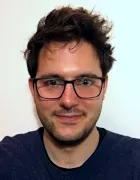
Julian Mayer-Steudte
Theoretical Particle and Nuclear Physics
Theoretical Particle Physics, Computational Physics
Supervisor: Prof. Dr. Nora Brambilla
Supported by: MDSI

Technical Physics
Controlling Patterns with a Spatial Light Modulator During Si Electrodissolution
Supervisor: Prof. Dr. Katharina Krischer
Supported by: Linde
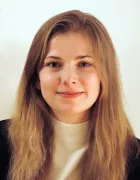
Data Analytics and Machine Learning
Robustness of Neural Networks for Combinatorial Optimization
Supervisor: Prof. Dr. Stephan Günnemann
Supported by: Linde
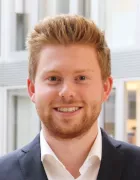
Mathematical Statistics
Identifiability and Inference of Causal Effects in Latent Variable Models
Supervisor: Prof. Dr. Mathias Drton
Supported by: MDSI
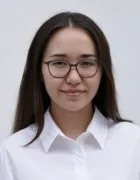
Kamilia Zaripova
Computer Aided Medical Procedures & Augmented Reality
Geometric Deep Learning
Supervisor: Prof. Dr. Nassir Navab
Supported by: Linde