Analyzing the goodness of fit of Regression Models
This project took place in summer term 2021, you CAN NOT apply to this project anymore!
Results of this project are explained in detail in the final report and presentation.
- Sponsored by: msg life
- Project Lead: Dr. Ricardo Acevedo Cabra
- Scientific Lead: Kilian Wohlleben
- TUM Co-Mentor: PhD Candidate Marija Tepegjozova
- Term: Summer semester 2021
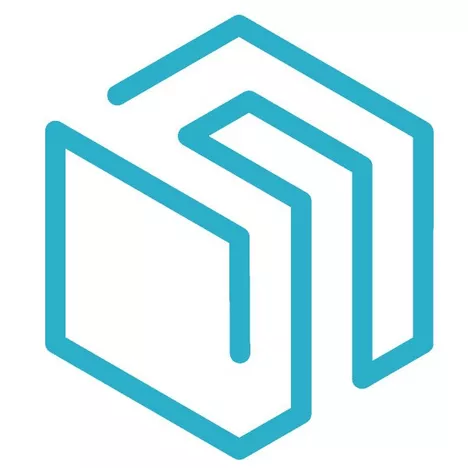
Do you want to learn about advanced statistical evaluation techniques? Do you want to work in a research like environment and develop your own ideas independently to unleash your full creativity? Do you want to be an important part of a multi-million Euro endeavor? Then this project might be something for you.
As the leading life insurance software provider in the DACH region, msg life is often faced with regulatory obligations, such as guaranteeing that our software solutions are up to the highest standard of accuracy. Currently we are developing methods to substitute the calculation of insurance premiums and other related values with neural networks.
As we are currently beginning to utilize machine learning methods in our products, we need to provide proofs that these standards can be met and that our models are in no way inferior to classical approaches. This poses several challenges, as neural networks are “black-box” models that don’t provide any guarantees for out of sample predictions.
Fortunately for us the sample space of our problem is known and finite but still too large to test every possible combination. Therefore we need to find other ways to prove the quality of our models over the entire sample space; not just a test set. These ways can be empirical, statistical or mathematical (check out the video for examples of what we mean by that).
The goal of this project is going to be to find ways to measure the goodness of fit over the entire sample space and visualize them for several existing models. This involves a systematic comparison of models, defining measures for the goodness of fit and testing methodologies. If possible you will also analyze the models itself to find guarantees that the model is reasonable from an actuarial point of view, however no experience in this area is required.
Accepted students to this project should attend (unless they have proven knowledge) online workshops at the LRZ from 06.04.2021 - 09.04.2021 (9:00 AM to 5:00 PM). More information will be provided to students accepted to this project.