Deep Learning on High-Res Multispectral Aerial Imagery
- Sponsored by: Ocell
- DI Incubator: Startup Ocell
- Project Leader: Dr. Ricardo Acevedo Cabra
- Scientific Lead: M.Sc. David Dohmen, M.Sc. Felix Horvat
- Term: Winter semester 2019
Results of this project are explained in detail in the final documentation and presentation.
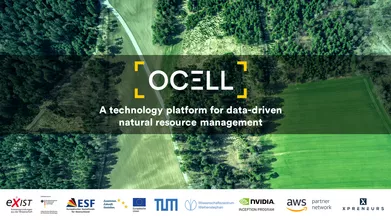
With 11.3 million hectares, a third of the German surface area is covered by managed forests. They all require extensive maintenance due to their ecologic and economic relevancy in our society. Changing climatic conditions bring difficult challenges to forest managers worldwide. Warmer and dryer summers have trees struggle with bark beetle infections. For instance, in 2018 the financial loss through bark beetle damages in Bavaria alone was higher than 100 Million Euros.
Stronger storms, snowfall and more tree diseases are further issues, our forests are facing nowadays. In such an endangered state, the work of forest managers is becoming more and more critical in order to preserve these important ecosystems and CO2-sinks. Periodic forest stock takings and quick assessments of damages are necessary but hard to achieve, due to the forests’ sizes and difficult accessibilities. In a typical forest stock taking forest workers travers the forests by food and take certain measurements at sample points, which are spread in a 100m x 100m grid all over the Germany. They count and specify trees, measure heights and thickness and assess damages. From these relatively sparse sample points, the results get interpolated, which is a statistically questionable step for complex mixed forests.
OCELL helps forest managers by digitizing many of these processes and providing a better data- and knowledge foundation for decision making. We generate aerial images of large areas such as forests with ultralight aircrafts and interpret the data with machine-learning approaches. With our specialized camera module, we generate high quality aerial imagery in five spectral bands at a resolution that has 600x more pixels per area than the best available satellites and doesn’t suffer from cloud occlusion, as we fly underneath them. This kind of high-quality data is necessary to extract meaningful information for forest owners. We generated several hundred gigabytes of multispectral imagery data of forests, from which we extract 3D-information through photogrammetric processing. This allows us to extract tree sizes and heights. In order to classify tree species, we develop convolutional neural networks, which work right on the georeferenced aerial imagery. Our five different spectral bands contain more information than the typical RGB bands, which allows a better separation of different tree species. Using a scientific database of tree parameters with over 450.000 trees, we can then apply regression models for relevant tree parameters like underground/ overground biomass and usable wood mass.
Lastly the multispectral imagery allows insights into chlorophyll contents and general plant vigor, giving us a chance to assess tree health and even detect infections when there’s still time to contain the issue.
All these findings and approaches build a cohesive picture of the forest’s state in order to support forest managers. Together with our pilot customers we iteratively audit our models to gain feedback, improve results and add useful features. We’re looking forward to work together with you on this fascinating topic!