Interpretable AI for business applications
- Sponsored by: Steering Lab by Horváth & Partners GmbH
- Project Leader: Dr. Ricardo Acevedo Cabra
- Scientific Lead: Dr. Andrei Belitski, M.Sc. Olena Schüssler
- Term: Winter semester 2019
Results of this project are explained in detail in the final documentation and presentation.
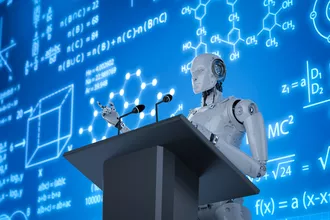
Food for inspiration on the proposed topic:
- Think of robots learning new behavior that need to explain it to a human what they have learned
- Think of machine learning algorithms that discover patterns in high-stake decision relevant data that in turn need to be unambiguously communicated to the executives making the decision
- Think of models that have to produce natural language output to explain relations or associations learned from unstructured data sources to its users
- ...
With the very current rise of ‘blackbox’ models such as the majority of models built on deep learning algorithms, interpretability of the results from these models lacks far behind, still ‘blackbox’ algorithms are starting to be employed in high-stake decision making in some domains. On the other hand acceptance and adoption of intelligent models within high-stake business environments more than ever crucially depends on model inferences being transparent and reasonably explainable for the target stakeholders. This is mainly due to facilitating the ability of informed decision-making, safety concerns, regulatory requirements, risk management, psychological trust - just to name a few dimensions.
Project Goal: In this project we are aiming to achieve explicit and humanly interpretable representation from algorithmically learned patterns by means of state-of-the-art methodology. The initial version of the model aimed to be the output of this project should prove its feasibility for one of the application domains of high interest to our clients.