Benchmarking Matrix for Automated Machine Learning
This project took place in summer term 2021, you CAN NOT apply to this project anymore!
Results of this project are explained in detail in the final presentation.
- Sponsored by: PwC
- Project Lead: Dr. Ricardo Acevedo Cabra
- Scientific Lead: M.Sc. Andrea Kulanthavadivel
- TUM Co-Mentor: PhD Candidate Leonardo Araujo
- Term: Summer semester 2021
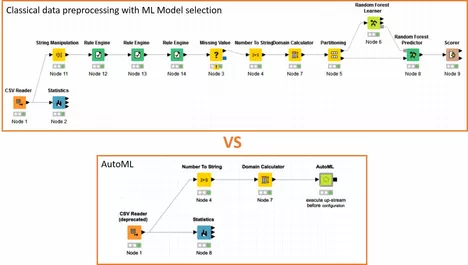
PwC has more than 276,000 employees worldwide at all the major business locations. We are growing globally, but also in Germany, where we are the auditing and consulting company with the highest turnover of €2.2 billion but we’re also the most attractive employer among the so- called “Big 4”. In Germany alone, approximately 11,809 employees are currently working at 21 attractive locations. On the one hand, this always puts us near our clients, wherever they are in Germany. On the other hand, this allows us to offer our (potential) employees a high degree of local flexibility, for example, when joining the company or during their careers at PwC. For more than 35,000 clients, we are an expert partner and a good advisor.
Our goal is to provide our clients with the services they request in an integrated way, ie, across all business fields. Our clients more often ask for easier ways to apply machine learning and data analytics on their data without the explicit need of an advanced Data Scientist. Automated machine learning is a common approach that could be applied for faster ML solutions by someone who has some knowledge in Data Science and therefore becoming more powerful in the industry.
Automated machine learning (AutoML) is the process of automating the end-to-end process of applying machine learning to real-world problems. In a typical machine learning application, experts must apply the appropriate methods of data preprocessing, feature engineering, feature extraction, and feature selection to make the data set usable for machine learning. Following these preprocessing steps, practitioners must then perform algorithm selection and hyperparameter optimization to maximize the predictive performance of the final machine learning model.
AutoML was developed as an artificial intelligence-based solution to the ever-growing challenge of applying machine learning. Automating the end-to-end process of applying machine learning offers the benefits of producing simpler solutions, faster creation of those solutions, and models that quite often perform better than models designed by hand.
The goal within the project is to:
-
Research on existing automated machine learning tools
-
Selection of the top five automated machine learning tools
-
Apply all five tools on the same industrial open source dataset for predictive analytics
-
Develop a benchmarking matrix
-
Demonstration of the results (e.g. via a demonstration video)
Data Source for the project will be an open source dataset.
Accepted students to this project should attend (unless they have proven knowledge) online workshops at the LRZ from 06.04.2021 - 09.04.2021 (9:00 AM to 5:00 PM). More information will be provided to students accepted to this project.