A robust comparison of causal effects from observational data in healthcare
This project took place in summer term 2021, you CAN NOT apply to this project anymore!
Results of this project are explained in detail in the final report.
- Sponsored by: Helmholtz AI and the Institute for Computational Biology
- Project Lead: Dr. Ricardo Acevedo Cabra
- Scientific Lead: Dr. Niki Kilbertus and Dr. Narges Ahmidi
- TUM Co-Mentor: PhD candidates Konstantin Göbler and David Strieder
- Term: Summer semester 2021
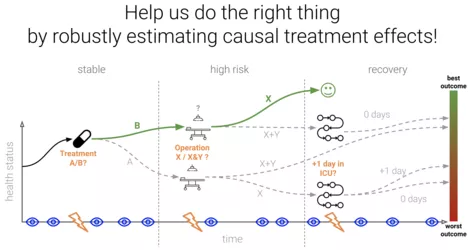
Goal: Quantify the direct or --- in the case of mediation analysis --- indirect causal effect of various treatment and medication decisions on adverse effects of patients both from realistically simulated as well as real clinical data.
Background: Machine learning has celebrated tremendous successes in pattern matching in a broad variety of domains, often allowing for astounding predictive accuracy. However, in the standard setting, these systems can only identify association, not causation. For example, there is a statistically significant correlation between chocolate consumption per capita and the number of Nobel prizes won across European countries (Messerli 2012). However, enforcing higher chocolate consumption may not actually drive up the number of scientific breakthroughs. Similarly, machine learning systems may easily identify statistically significant dependencies between certain lifestyle choices such as the daily time spent reading or certain diets and the severity of disease progression when contracting COVID. How should we decide which factors are worth actively changing and which are merely correlated via other factors. Perhaps the same people who read a lot tend to lead healthier lifestyles in general. These effects lead to important questions in health-care: When having chosen one of multiple possible treatments, how can we show that it was actually the treatment that cured people? More broadly, how can we assess and compare causal effects in complex biological systems where we do not perfectly understand the underlying processes?
Methods: To answer this question, we will make use of various methods in the causal inference toolbox (Pearl 2009; Peters, Janzing, and Schölkopf 2017). As a first step, to answer causal queries, we will have to make reasonable assumptions about the causal structure of the variables included in our simulated clinical dataset. We will then develop machine learning models that are able to quantify direct and mediated causal effects while taking into account possible confounding factors that influence both the treatment as well as the mortality.
Data: To get a feeling and properly understand and assess our causal inference tools, we will start out working on simulated clinical data with known ground truth behavior. This database closely mimics all characteristics of actual patient data, without posing any risk of privacy leakage. Then, we will move to real healthcare data related to a large study of roughly 2000 patients who received stent implants. The data contains about 78 covariates about demographics, medication, risk factors, procedural information, adverse event reports and eventually follow up assessments. Within this dataset, the goal of the project will be to robustly estimate the direct and indirect causal effects of certain treatment decisions as well as predispositions on the overall outcome of the operation.
Accepted students to this project should attend (unless they have proven knowledge) online workshops at the LRZ from 06.04.2021 - 09.04.2021 (9:00 AM to 5:00 PM). More information will be provided to students accepted to this project.
References
Messerli, Franz H. 2012. “Chocolate Consumption, Cognitive Function, and Nobel Laureates.” The New England Journal of Medicine 367 (16): 1562–64. Pearl, Judea. 2009. Causality. Cambridge University Press.
Peters, Jonas, Dominik Janzing, and Bernhard Schölkopf. 2017. Elements of Causal Inference: Foundations and Learning Algorithms. MIT Press.