Uncertainty Estimation for Deep Medical Image Segmentation
This project was for summer term 2020, you CAN NOT apply to this project anymore!
- Sponsored by: Carl Zeiss AG
- Project Lead: Dr. Ricardo Acevedo Cabra
- Scientific Lead: Dr. Ghazal Ghazaei and Dr. Alexander Urich
- Co-Mentor: Dr. Tobias Köppl
- Term: Summer semester 2020
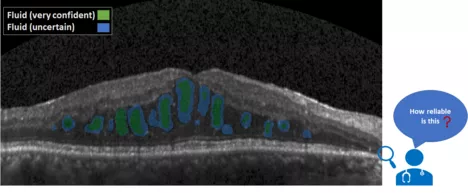
Results of this project are explained in detail in the final documentation and presentation.
ZEISS Who? With more than 25,000 employees, ZEISS is one of the global leaders in the optical and optoelectronic industries and has been contributing to technological progress for 170 years. ZEISS develops and distributes lithography optics, measuring technology, microscopes, medical technology, eyeglass lenses, camera and cine lenses, binoculars and planetarium technology.
ML and ZEISS? As the machine learning and computer vision team of ZEISS corporate research and technology, we concentrate on cutting edge machine learning solutions for the vast variety of applications at ZEISS. Being one of the pioneers in development of ophthalmology devices, we are keen to apply our methods to ophthalmic images and contribute to enhancement of medical care for patients suffering from eye diseases.
Cool - and healthcare? In ophthalmology, Optical Coherence Tomography (OCT) plays a key role in diagnosis as a non-invasive modality thanks to providing the capability of visualizing retinal patterns in cross-sectional images. Not only OCT images are of great importance for early diagnosis of numerous pathologies, but they also can illustrate tissue functionalities, blood flow and oxygenation for deeper analysis of a present abnormality. As such, several OCT datasets are available to study various retinal pathologies via segmentation and classification of these images.
So – ML for healthcare! What’s left? The goal of this project is to automatically provide an accurate segmentation for OCT images captured from the retina. For example, a common use-case in ophthalmology is to analyze the size of fluid-filled areas in the eye (e.g., intraretinal fluid (IRF), subretinal fluid (SRF), or pigment epithelial detachment (PED)) which helps medical experts in their diagnosis. This is a simple task for Deep ConvNets, right? But would you trust this black box? Should a plain CNN prediction be the basis for a doctor’s treatment?
Therefore, we will go beyond standard segmentation in this project and investigate how to predict uncertainty maps reliably. Thereby, doctors are informed of the regions of interest as well as about the model’s uncertainty in those regions. This added value can help doctors in their decisions while easing the introduction of machine learning solutions into medicine.