Prediction and Clustering Critical Suppliers
This project took place in summer term 2022, you CAN NOT apply to this project anymore!
Results of this project are explained in detail in the final report.
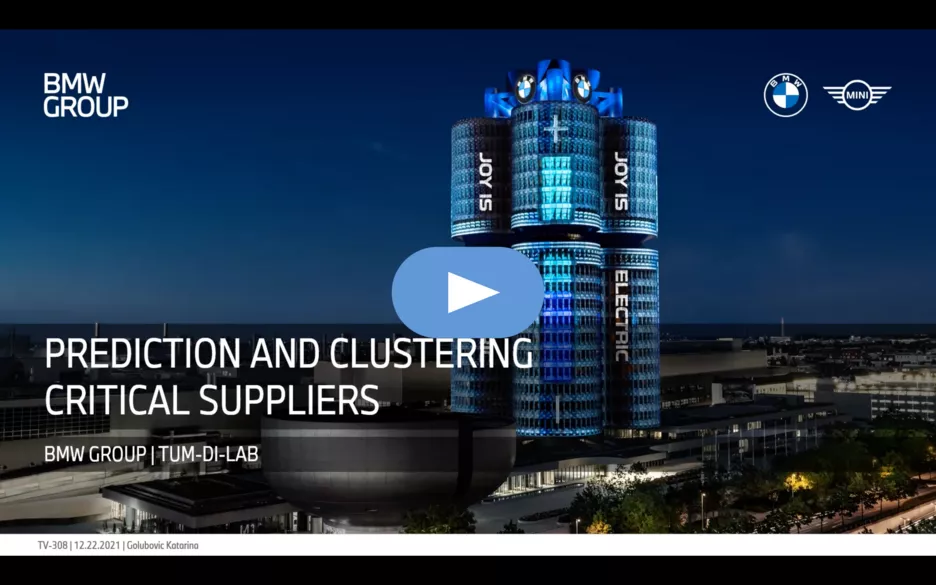
- Sponsored by: BMW Group
- Project Lead: Dr. Ricardo Acevedo Cabra
- Scientific Lead: Sergio Correa, Ishansh Gupta, Katarina Golubovic.
- TUM Co-Mentor: PhD candidate Jun Wu
- Term: Summer semester 2022
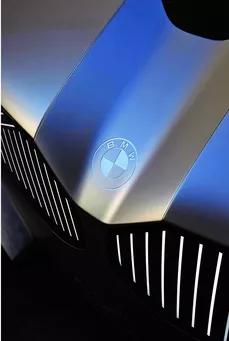
Think Tank TV-308 BMW Group
We are part of the BMW digitalization group in supply chain management. Our goal is to deliver assurance by making sure that our plants have enough required parts to produce cars on time. Daily we analyze and report critical suppliers within the BMW Group. Our goal is to use data-driven models, to detect, predict and cluster our critical suppliers. This way we can optimize the allocation of our current stock, be prepared for upcoming changes, and work only with reliable suppliers.
Make a difference!
Recent years have proven that supply chains have become more challenging to predict and manage. The increasing complexity of flows and market volatility, which had been exacerbated by the COVID-19 pandemic, have elevated the need for agility and flexibility. Supply chain management solutions based on artificial intelligence (AI) are expected to be potential instruments to help organizations tackle current challenges. AI’s ability to analyze huge volumes of data, understand relationships, provide visibility into operations, and support better decision-making makes AI a potential game-changer. Digitalization and AI technologies will enable end-to-end transparency in the supply chain and faster decision-making.
This project focuses on understanding the connection between features of suppliers and using them to predict and cluster critical suppliers. Every supplier has features that define his current ranking. Features are captured on a daily/weekly basis and include indicators for wrong deliveries, backlog, missing parts, etc. At the moment supplier ranking is defined as a simple average of different features. Our goal is to use a machine learning approach to provide innovative metrics for identifying and predicting critical suppliers in the future.
The project is organized in the following steps. First, compare and identify the main features that define the supplier. Understand and analyze historical data. Second, train a time series algorithm that will predict the values of given features. Third, classify suppliers to identify and predict an escalation in the future. Finally, evaluation of given models and model explainability so that data decisions are transparent.
We are looking for creative and self-driven candidates. Bringing your ideas and extending the scope is something that is highly valued.
Accepted students to this project should attend (unless they have proven knowledge) online workshops at the LRZ from 19.04. - 22.04.22. More information will be provided to students accepted to this project.