Explainable AI Applied to Dynamic Credit Risk Models
Results of this project are shown in the demo video and the final report (PDF) below:
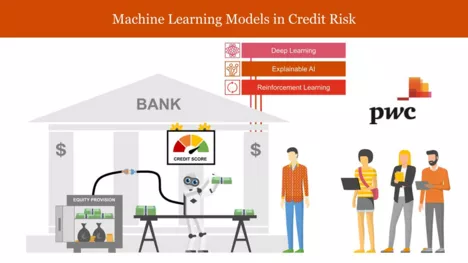
- Sponsored by: PwC
- Project Lead: Dr. Ricardo Acevedo Cabra
- Scientific Lead: Oliver Kobsik, Stephan Bautz, Sophie Mutze, Jan-Patrick Schulz
- TUM Co-Mentor: Prof. Massimo Fornasier
- Term: Winter semester 2023
- Application deadline: Sunday 23.07.2023
About PwC
The PwC network spans the globe and employs nearly 300,000 people. As part of the global PwC Network, PwC Germany is well-established in this country having 21 locations and 13.000 employees while we are constantly on the lookout for new colleagues who are enthusiastic about innovation. Everyday PwC Germany is confronted with new challenges and issues, which we always address with our values in mind: We act with integrity, we make a difference, we work together, we care for others, and we reimagine the possible day by day.
In our subcluster 'CDO' in Financial Services Technology Consulting, we advise our clients - multinational banks, insurance companies, and finance-oriented corporates - on the digital transformation of their projects. This includes the areas of Data & Analytics, Big Data, Artificial Intelligence, Blockchain Technologies, and Credit Risk.
About the project
Are you interested in predicting the Probability of Default with an AI model that can replace traditional models and meet regulatory requirements with explainable AI?
Institutions like banks and insurances spend thousands of hours building regulatory-compliant credit risk models like PD (Probability of Default), LGD (Loss Given Default), or EaD (Exposure at Default). At the end of the day, a handful of risk drivers are included in the final model. These risk drivers are typically selected with a linear or logistic regression. These regression models involve the use of an optimization algorithm to find a set of risk drivers for each input to the model that minimizes the prediction error. The model performance is evaluated using measures like Somers'D (Gini-Coefficient). Before calculating this value, data transformation steps are performed to enhance data stability and comparability. Highly correlated risk drivers should not be included in the model at the same time. Machine Learning algorithms should be expected to recognize such correlations and even find interrelationships that cannot be found with the traditional statistical approach.
To address this problem in case of a PD model, we utilize a historical dataset containing risk drivers and a 'Default Flag' column indicating entity defaults at a specific point in time. Based on this information, we develop a deep learning network to establish a model and assign the Probability of Default to each entity in the dataset.
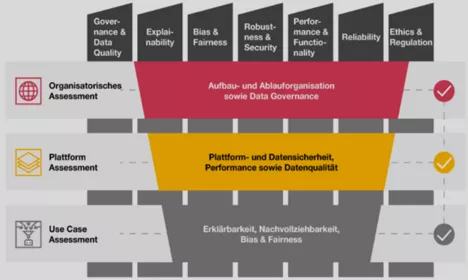
Now the exciting part begins. We want to find out why the developed AI decided the way it did. For this, PwC already developed a solution. You can take our information and make add-ons, or you can develop a new explainable AI tool from scratch.
Additionally, we aim to make the model dynamic, as recent events such as COVID-19, wars, or bank collapses have shown that semi-annual or annual model updates may not be sufficient to meet our expectations. Therefore, we propose incorporating reinforcement learning techniques into the model to enable it to respond promptly to data changes and remain effective in a rapidly evolving environment.
Objective:
Our goal is to anticipate the coming trends by bringing AI-driven solutions to the regulatory market and replacing the inflexible traditional statistical models. Once this happens, a new decade for regulation will begin and represent a major shift for the further integration of AI solutions.