End-to-end process enhancement for Crew management
This project took place in winter term 2020, you CAN NOT apply to this project anymore!
Results of this project are explained in detail in the final documentation
- Sponsored by: Celonis & Lufthansa CityLine
- Project Lead: Dr. Ricardo Acevedo Cabra
- Scientific Lead: Dipl.-Ing. Philipp Grindemann (Lufthansa) and M.Sc. Janina Nakladal (Celonis)
- TUM Co-Mentor: PhD candidate Leonardo Araujo
- Term: Winter semester 2020
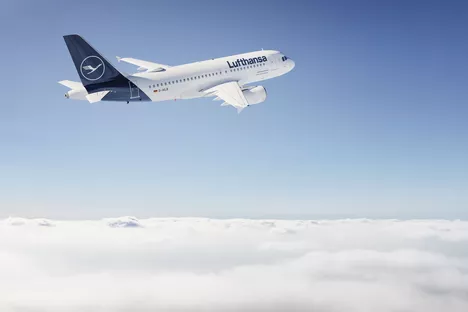
Motivation and Value Proposition
- Our employees are the most valuable assets of our company
- Therefore crew management is one of our key processes, as the process ensures that the right people are on the right flight at the right time
- Irregularities, like lack of crew causes flight cancellations or delays
- Ineffective crew planning leads to unproductive crews and imbalanced utilization
- Currently crew management and crew staffing is based on expert knowledge, human decision making and sub-process optimization
- The next step is "end-to-end" transparency and a holistic optimum, from capacity planning to the actual flight event
- Improved crew processes lead to a higher utilization of crews, better prediction of deviations and reduction of process cost
- Process prediction and data driven decision support for crew planners reduces complexity, rework and effort in crew management
- Improved process handling plays well with Lufthansa CityLine’s continuous improvement program and other process improvement initiatives
- Advanced process steering reduces need for standby crews and improves utilization, fair crew schedules and crew happiness
Content / Deliverables
- Process analysis: As-is analyses of the current process and implications on crew staffing, standby planning and productivity of crews.
- Process prediction: Design and implementation of a prediction model / steering triggers for the crew management process: identify proper data points, link process and master data, choose and implement proper prediction algorithms / triggers (e.g. clustering – freely selectable) .
- Process steering: Define measures to avoid process deviations collaboratively with business areas and implement proper monitoring of measures.
- Operations: For all three topics, keep maintainability, performance and scalability in mind; the prediction model must be adaptable to more staff.