Federated Learning
Collaborative machine learning without centralized training data
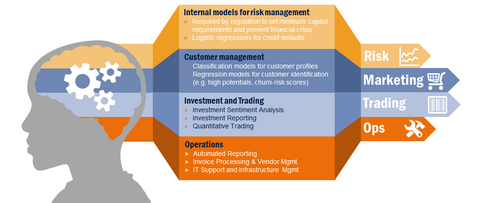
- Sponsored by: d-fine GmbH
- Scientific Lead: M. Sc. Todor Dobrikov
- Project Lead: Dr. Ricardo Acevedo Cabra
- Term: Summer semester 2018
Machine learning techniques make it possible for companies to gain a better and deeper insight into their customers’ data. Modern financial institutions are no exceptions. Often it is more feasible to cooperate and develop a common model instead of building an individual solution. Joining forces and combining expertise greatly reduces development and maintenance costs. Additionally, the own data to train and validate a model which is an important premise for good results is often not sufficient for a good model performance. The complexity in the collaborative development (and validation) of joint models arises from the fact that customer data are highly sensitive and valuable to the individual institutions and thus they are not willing or even allowed to share them. Federated learning enables the companies to benefit from the higher amount of available data without exposing them to their competitors by training individual models and combining them to a joint model with a lot higher accuracy. For more information please read the document and contact M.Sc. Todor Dobrikov.
Results: The results of this project were summarised in the presentation and explained in detail in the final documentation.