Resource Forecasting for Satellite Operations using Multivariate Time Series Data
This project took place in winter term 2020, you CAN NOT apply to this project anymore!
Results of this project are explained in detail in the final documentation and presentation.
- Sponsored by: German Aerospace Center (DLR)
- Project Lead: Dr. Ricardo Acevedo Cabra
- Scientific Lead: Dr. Clemens Schefels and M.Sc. Leonard Schlag
- TUM Co-Mentor: PhD candidate M.Sc. Philippe Sünnen
- Term: Winter semester 2020
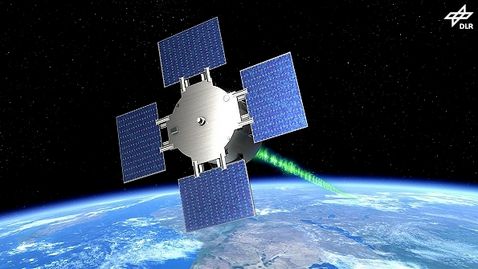
© CC-BY 3.0
Most of the resources of a satellite, once launched into space, are very limited and have to be shared with all on-board consumers. For example, the current power is mostly produced by small solar panels. The power output of these panels restrict the on-line time of power consuming equipment like cameras or radio devices. Therefore, one main job of satellites operators is to plan the tasks of a satellite in respect to its available resources. As real-time data is only available for a few minutes every couple of hours for most LEO (Low Earth Orbit) satellites, a dynamic and generic means of prediction of resources could greatly aid operations.
The German Space Operations Center (GSOC) at the German Aerospace Center (DLR) commands a fleet of modern satellites, each with thousands of on-board sensors to keep track of satellites system status. Many of the satellites are in routine-phase, allowing us to accumulate a sufficiently large training set for machine learning purposes.
The goal of this project is to forecast a single parameter, e.g., a battery voltage, using all related parameters and planned commands which are to be executed on the satellite as input. The forecast horizon should at least be a couple of hours. This means, to investigate and compare different methods for multivariate time series forecasting, i.e., supervised/semi-supervised ML techniques such as regression, neural networks, random forests, etc. The result is an improved resource planning with greater efficiency and a possible enhancement of the current mission planning algorithms. Launch your mission with us!