Machine learning-based image detection for lensless microscopy in life science
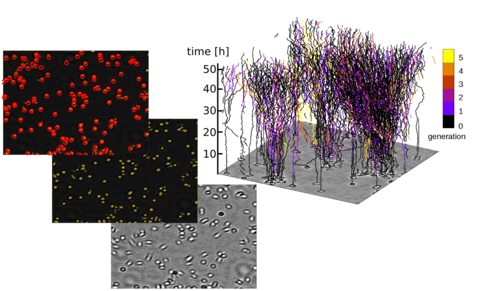
- Sponsored by: Soft condensed matter group. Faculty of Physics, LMU
- DI Incubator: Potential Startup
- Project Lead: Dr. Ricardo Acevedo Cabra
- Scientific Lead: Dr. Philipp Paulitschke, Dr. Korbinian Paul-Yuan
- Term: Summer semester 2019
Results of this project are explained in detail in the final documentation and presentation.
Our research group develops innovative and scalable imaging solutions for cell-biological research such as in vitro experiments with cultured cells. These experiments are important to study the growth and migration patterns and allow to gain a better understanding of cancer progression and helps to develop better and more effective cancer-treatments.
We have developed a fast and super small digital holography lensless microscope (LM) that can be universially applied and easily integrated in life science labs. Recently, we have proven that our LM, equipped with smart image detection algorithms (deep convolutional neural networks and residual learning) can detect the single cell traces of cultured cells with the same quality than high resolution microscopy, which is 10 times bigger and way more expensive. Therefore, LM and smart image detection yield a framework to continuously monitor vital parameters of cultured cells down to the level of single cells.
The goal of this project is to extend this framework with a detector that quantifies other important cell vital parameter to make the LM a universal platform technology. Therefore, we have prepared high-quality annotated datasets for supervised training of the networks and benchmarking. The result of this project will have direct impact in cell-biological labs, facilitating the work of cell biologists and will accelerate the foundation of the planed start-up, financed by “Exist-Forschungstransfer, Bundesminsterium für Wirtschaft und Energie”.