Deep learning for genetic risk prediction
This project was for summer term 2020, you CAN NOT apply to this project anymore!
- Sponsored by: Institute of Computational Biology, Helmholtz Zentrum München
- Project Lead: Dr. Ricardo Acevedo Cabra
- Scientific Lead: Dr. Matthias Heinig
- Co-Mentor: M.Sc. Michael Rauchensteiner
- Term: Summer semester 2020
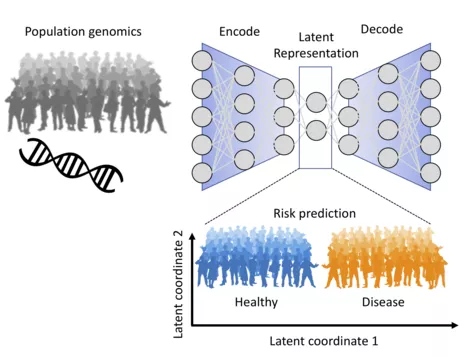
Goal: Leverage current developments in deep learning to predict genetic risk for common diseases.
Background: Deep learning has been very successful in image processing, where large bodies of training data (N > 10^6) are available. In the domain of molecular biology deep neural networks have been applied to classify functional regions (N ~ 10^5) of the genome based on DNA sequence (for review see Eraslan et al. 2019) or to describe cell states of single cells (10^4 < N < 10^5) based on their RNA expression profiles. Deep generative models such as variational autoencoders allow for learning latent representations of the expression profiles (Lopez et al. 2018) that can be used to predict cellular response (Lotfollahi et al. 2019). Here we hypothesize that this approach would also be able to learn meaningful latent representations from genetic data of the general population. We would like to show that these latent representations allow for an improved prediction of disease risk for a variety of common diseases compared to current polygenic risk scores. However, in genetic data the number of individuals is usually much lower (10^4 < N < 10^5) than the number of observations (p ~ 10^6) and therefore also the number of model parameters that have to be estimated.
Methods: To tackle the p>>N problem, we propose to make use of prior information on the impact of genetic variants on RNA abundance (Gusev et al. 2016). We will explore how this prior can be encoded into the network architecture. This network will be part of a variational autoencoder for learning a latent representation of the general population, which comprises many individuals at risk to develop common diseases. In the second step we will explore how the occurrence of common diseases relates to the latent variables and whether they can be used for risk prediction. These predictions will be compared to state of the art polygenic risk scores.
Data: We want to use the UK biobank, which is the world’s largest population cohort (N = 500,000) with genotype data available. We will focus on risk prediction on prevalent common diseases such as coronary artery disease, diabetes and others. In addition we also have a large in-house database with genetic data and health status available for validation.
References: Gusev et al. (2016). Integrative approaches for large-scale transcriptome-wide association studies. Nature Genetics Lopez et al. (2018). Deep generative modeling for single-cell transcriptomics. Nature Methods Lotfollahi et al. (2019). scGen predicts single-cell perturbation responses. Nature Methods