ML-Driven Time Series Analysis for Predictive Maintenance of Wind Turbines
- Sponsored by: BayWa r.e.
- Project lead: Dr. Ricardo Acevedo Cabra
- Scientific lead: Milena Zurmühl
- TUM co-mentor: Jona Klemenc
- Term: Summer semester 2025
- Application deadline: Sunday 19.01.2025
Apply to this project here
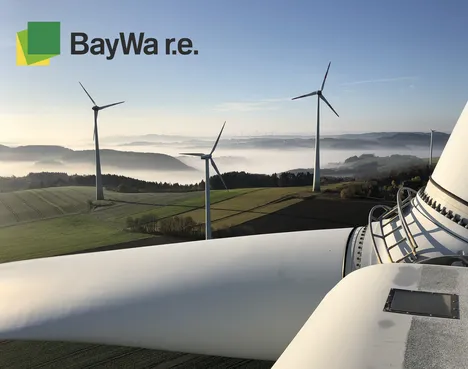
About BayWa r.e.
BayWa r.e. is the home for change makers. We r.e.think energy – how it is produced, stored and can be best used to enable the global renewable energy transition that is essential to the future of our planet. At BayWa r.e. we effect change globally, being a leading global developer, service provider, distributer, and solutions provider. With a focus on wind and solar energy, we have, so far, brought over 6 GW of energy online and manage over 10 GW of assets.
About the Project
Besides building new power plants, optimizing the management of existing energy projects is essential. It improves efficiency, reduces costs, and extends their lifespan, enhancing their contribution to the energy transition. At BayWa r.e., we achieve this by leveraging real-time data monitoring, collecting extensive historical data, and developing advanced analytics that unlock valuable and actionable insights.
In this project, we aim in particular to enhance the management of wind farms, by applying advanced data analytics for evaluating the technical health of individual turbine systems, thus enabling predictive maintenance. Analyzing time series data from several temperature and pressure sensors of turbine components, as well as results from regular oil inspections, the objective is to define dynamic thresholds that can be used as an evaluation criterion for the turbines’ condition. To achieve this, our main focus lays on the detection of signal-specific anomalies in the historic data. The respective algorithms should thereby be designed to identify both, individual outliers as well as abnormal patterns or trends over time. Recognizing the inherent dependence of the components’ temperature and pressure values on the turbines operating state, the analysis will also incorporate operational time series signals to better interpret the data and tailor the dynamic thresholds to the operational context. This way we aim to establish a robust evaluation framework for identifying deviations that may indicate potential issues. To enable a more nuanced assessment of those deviations as an indicator of future problems, the goal is to further categorize the detected anomalies into clusters and cross-reference them with a dataset of known turbine failures. This allows for the calculation of cluster-specific failure probabilities, adding valuable insights into the criticality of the turbine’s condition. The resulting data-driven evaluations will then provide a solid foundation for predictive maintenance strategies, enabling early decision-making and empowering project managers to take proactive maintenance actions. Consequently unexpected downtimes of turbines can be reduced significantly enhancing the overall operational efficiency of wind farms.
The data foundation for this project of technical health evaluation of wind turbines, consists mainly of telemetry data, including both, operational data as well as specific sensor measurements. The dataset encompasses historical data from a total of over 900 wind turbines, which were gathered over the last 5 years with a time resolution of 10 min. The results from the oil inspections are collected in a half yearly interval and will be provided for the same period of time. The dataset of known turbine failures, which is needed for the criticality estimation of detected data deviations, is based in turbine specific status data which is continuously collected and also available for the last 5 years. All relevant data is hence been provided in a structured way. Nevertheless, supplementary Natural Language Processing (NLP) techniques may be required, to extract additional insights from various text sources such as additional inspections reports.
Targeting a cloud-based solution, from a technical perspective, this project provides the great opportunity to engage with the latest state-of-the-art platform technologies, specifically Azure Databricks. This analytics platform was optimized for the Microsoft Azure cloud services and facilitates the seamless integration of data analytics, machine learning workflows, and scalable computing resources, ensuring an efficient processing and analysis of the extensive time series dataset of wind turbines.
We are looking forward to your application.
Apply to this project here